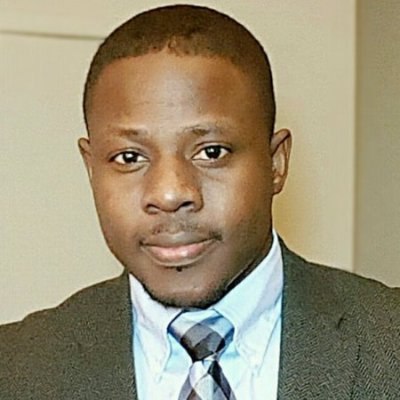
Paper: Uncertainty Characterization in Quantitative Models
Abstract: The treatment of uncertainty quantification has evolved over the years. One of the main drivers of this sweeping paradigm shift has been the advances in computing power. Today, with the exponential advances in computing power relative to the past, we can collect an unprecedented amount of data over an almost unlimited period of time. Big Data, as folks in the statistical analytics field like to call it, has expanded past the barriers of previous limitations of data analytics. But there’s a problem; our over-reliance on computer analytics and our insatiable appetite to ‘play with the numbers’ means more often than not, we cannot spot mistakes within our computer models and blindly follow what the numbers say. As powerful as computers are, and as advanced as analytics platforms have become, quantitative models perform optimally when guided by expert intuition. These computer based models grapple not only with physical variabilities in the real system they emulate, but also with potential deficiencies within the model itself due to a lack of knowledge about the system being modeled or its surrounding environment. Today, every industry seeking a competitive edge is using data to shape its decision making under uncertainty in one way or the other. This paper, following recent advances in uncertainty quantification in the leading domains of engineering, statistics and climate change, presents the current state of the practice within these domains. The paper also presents a synthesis of recent research.
Biography: Adiel Komey is a doctoral candidate in civil engineering at the University of Maryland and holds a BSCE from Kwame Nkrumah University of Science and Technology, Ghana. He has consulted widely in the hydropower and dams industry, including to Ontario Power Generation, DC Water and Sewer Authority, the US Army Corps of Engineers, and the Volta River Authority.